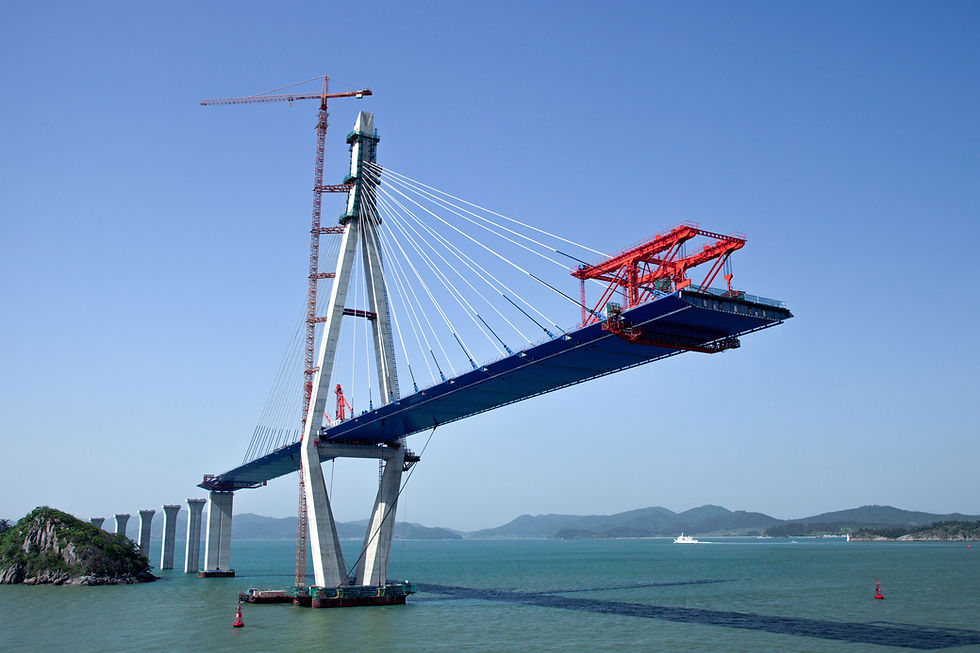
I recently had the opportunity to start an exciting greenfield project that involved creating a REST API capable of connecting to multiple LLMs. Since this is a greenfield project with no inherent language requirements, Python was my natural choice. All the major LLM platforms consider Python a first-order language and create native Python SDKs to interact with their APIs.
Fast API, Flask, and Django are the 3 Python frameworks I considered using to create the API. I have worked with each framework in the past and already have familiarity with each. Below are some of the key characteristics of each framework, followed by a feature comparison table.
Key Characteristics of FastAPI, Flask, and Django
FastAPI:
When to use: Your core use case is building a modern, documented API with great performance.
Designed specifically for API development
Modern (built on Python 3.6+)
Fastest of the three – uses Starlette to achieve high performance
Built-in support for asynchronous programming
Supports type checking and data validation with Pydantic
Automatic API documentation via OpenAPI Swagger
Easy to learn and use
Flask:
When to use: You need a micro-framework where flexibility/extensibility is critical and you want customization options in how your API is structured.
Highest degree of flexibility in terms of project structure and design choices
Lightweight and flexible micro-framework
Minimal core with easy extensibility
Large ecosystem of extensions
Easy to understand and get started
Suited well for both small and large projects
Django:
When to use: You want to build an API that is part of a larger, fully featured, full stack web-framework with an extensive toolkit and a built-in admin page.
Full-featured, full stack web framework with a built-in admin interface
Well-suited for large, complex projects that require a lot of built-in functionality
Follows the Model/View/Controller pattern
Comprehensive ecosystem with many built-in features
Strong security features baked into the framework
Large community with extensive third-party packages
Feature Comparison Chart
Below is a feature comparison chart I created that lists out some specific capabilitlies and tooling for each framework:
| FastAPI | Flask | Django |
Asynchronous | Yes | No | No |
Authentication | Options for FastAPI Users, OAuth2, JWT | No built-in auth system, extensions available | Robust and extensible |
Background Tasks | Built-in support | No, use Celery | No, use Celery |
Complexity | Low, easy to learn and use | Low, very flexible | High, steeper learning curve with all the built-in features |
Cross-Origin Resource Sharing (CORS) | Built-in CORS middleware | Can be added with Flask-CORS | Can be added with django-cors-headers |
Development Speed | Rapid, especially for API focused | Rapid, especially for small-to-medium projects | Slower initial setup, but rapid development for full-stack applications |
Logging | Standard Python logging | Standard Python logging | Built-in logging with extensive configuration |
Object-Relational Mapping (ORM) Support Built-In | No, use SQLAlchemy | No, use SQLAlchemy | Yes |
Optimal Environment | Microservices architecture | Highly customizable | Full stack development |
Request Validation | Built-in with Pydantic | No built-in validators, use Marshmallow | Provided via Django REST Framework serializers |
Security | Built-in security features | Basic, requires additional setup for comprehensive security | Robust security features out of the box |
Speed | Very high performance | Good performance, can be optimized | Good performance but generally slower |
Swagger Docs | Yes (Automatic) | No | No |
Web Sockets | Built-in support | No, use Flask-SocketIO | Build-in support via Django Channels |
Conclusion
For my project, I decided to go with FastAPI. It ended up being the perfect fit for my particular use case. The project's UI was built in Flutter/Dart, so there was no need for the full suite of capabilities provided out of the box with Django. I also didn't need the high degree of customization provided by Flask and decided to trade customization/extensibility for standardization, performance, automatic documentation, and access to asynchronous programming.
I hope this post distills some of the differences between the three frameworks and helps you in choosing the right framework for your next Python API project!
Comments